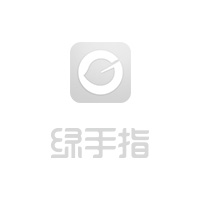

With technology sprawled in the society through screens, there can be no better role of stepping out than this. Walking through a park, climbing a mountain, or even relaxing in a garden provides number of positive effects on the human body, mind, and spirit. The above activities can help to break the monotony and also are very important in enhancing the health of a person.
Physical Health Benefits
Being an outdoor activity, most of the activities involved in this physical health improve are enjoyable. This is in contrast to indoor exercises that have to be performed indoors and may at times turn out to be very boring or monotonous leading to little action by the people involved. Brisk walking, running, cycling, swimming or playing football or tennis is excellent for a full body exercise that aid cardiovascular fitness, muscular endurance and elasticity.
Also, getting a chance to sunbathe while performing outdoor tasks helps in the intake of Vitamin D that is important for bone and the immune system. Another nutrient which is believed to cause various diseases if not adequately supplied in the right proportions in the body is Vitamin D, it is believed to cause osteoporosis, some types of cancer and mood swings. Staying outdoors also guarantees that the body gets enough sunlight to increase the Vitamin D in the body thus minimizing the incidence of such diseases.
Playing outdoors also contributes towards upholding a healthy weight of the body. Since most outdoor practices involve natural grounds, any activity that is done outdoors involves more energy expenditure compared to indoor surfaces. This extra effort helps in expending more calories hence aiding in the control of weight in the body. Besides, activities done outside are experienced to be physically demanding for longer and more rigorous periods because the natural environment acts as a form of entertainment that distracts people from the sweating they are undergoing.
Mental Health Benefits
It is actually found that the psychological benefits of outdoor exercises are perhaps just as much or even more than the physical benefits. Modern society can be characterized by developing stress levels, anxiety, and even depression, outdoor activities solve this problem on their own. There is a strong folklore that going for a walk, any kind of walk in the fresh air and where green trees or hills can be seen, can work wonders for the soul. It is for this reason that a simple act of getting out of doors, under the trees, near the water, or under the sky's vast canopy has been known to bring the mind to rest.
The ability of outdoor activities to lower concentration of cortisol, the stress hormone, is one of the outstanding benefits of outdoor activities for mental health. Of particular interest is the fact that when cortisol is high, then one is likely to experience anxiety, get depressed or even have a poor memory. It also reduces the level of cortisol thereby enhancing the general health of the brain.
Outdoor activities also help in increasing the level of mindfulness, a concept that demands that the mind dwell on the present moment with minimal criticism. Nature generally helps in making people pay attention to their surroundings through touching, seeing, hearing, etc NATURE IN THE PARK Bringing in nature itself will help encourage awareness through the use of our five senses. Such patience of mindfulness actually has the benefit which consists of reducing the possibility of stress, anxiety, and depression, increasing the ability to focus, and cultivating more appreciation and happiness.
Social Benefits
Outdoor activities can also present occasions to interact with people, and that is another component of welfare. Be it a family outing in a park, a team trekking or even a school sports day, the involvement in a sporting event can create better relations socially. Companion has a radical role playing in emotional health since they make the individual feel protected, valued, and have purpose in the society. Fun activities also create a sense of involvement in a team, communication, and building of proper interpersonal relationships.
As for children, outdoor activities are important in the overall process of their social human interaction. To support the key argument, it can be suggested that pre-school children playing clustered in an outdoor environment enhances their pro- social development skills including sharing, persuasive speaking and generative empathy. It also strengthens creativity and problem-solving as most children tend to come up with games or challenge that has to be met using the natural surrounding.
Connection to Nature
A very deep value that can be understood from the aspect of outdoor activities is the possibility to interact with nature. This link is not only a calming one but can also help a person or a community establish a relation with the World, thus making them a steward and take responsibility for the environment. There is hardly any doubt that nature has a gentling effect on the human spirit; it has the power to effectively reorient the mind to the different existential issues in life as well as reawakens the soul to the fact that there is a huge universe out there in which is are but small players.
Staying in natural areas can also make people become more considerate with the environment and, thus, live sustainably. A group of people who apply their leisure time for outdoor activities are generally aware of the possible negative effects they impose to the environment or the eco-system and would more likely fight for the conservation of such areas. It is pivotal to the health or the self as well as to the earth that this linking to nature occurs.
Boosting Creativity and Problem-Solving
Outdoor activities help in increasing creativity and problem solving as it has been evidenced among the adventurers. Constantly changing environment of the natural surroundings provokes the brain to work in different way and make connections that were not possible before. Hiking or camping, or any activity which may take place in the outdoors demands critical thinking and recourse to solutions.
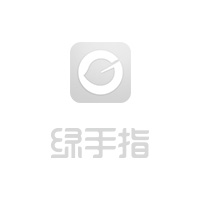

In the present generation, being able to reach out to other people is not only a nice thing to do but is rather necessary. To be informative, interpersonal skills could dictate the difference between clinching a job or not, between being accepted in a circle of friends or not or between ending up in a successful marriage or not. But what exactly does interpersonal skill means and how best can such a skill be developed?
Understanding Interpersonal Skills
Personal communication skills are sometimes called "interpersonal skills" and are, in essence, a broad set of competencies that are related to people interaction. Such skills are interpersonal skills particularly the oral and nonverbal communication, listening, understanding of perspectives when dealing with people, compassion, reconciliation, and cooperation. By cultivating such skills, one can be able to better relationship skills, improve job performance and hence lead a better life.
The Importance of Self-Awareness
The process of constructing proper interpersonal skills starts with personality examination. Knowledge of the self is important; it contains strengths, weaknesses, feelings, and behaviour towards different conditions. Self- awareness also makes one conscious of how his behavior impacts the others making one make more conscious attempts to change such behaviors.
To step up your self-awareness, begin by essaying a look at how you cope with people around you. Persuade your interlocutor to change his/her mind by the way you sit or stand, speak or gesture. Moreover, one might try asking friends, work colleagues or mentors to tell him or her how they experience that person. Such feedback can be very useful to understand potential of development.
Mastering Active Listening
Interpersonal communication entails a few measures, but one of the most vital is active listening. While considering active listening one must remember that it is more than just hearing the words that are told by someone; it also involves comprehending the message conveyed and even reacting to it. In other words, active listening is a way of gaining the other person's trust, admiring them and making the connection stronger.
Imagining is part of the active listening process, and to achieve this, whites the speaker is talking, try not to interrupt or even start framing an answer. These would go a long way in acknowledging the speaker and showing the audience that one is following what the speaker is saying by using non-verbal gestures like nodding and extending eye contact. Summarize the data or information that has been delivered by the speaker and parrot the words spoken by a speaker in order to check the level of understanding an unfamiliar language and to get rid of uncertainties.
In the present generation, being able to reach out to other people is not only a nice thing to do but is rather necessary. To be informative, interpersonal skills could dictate the difference between clinching a job or not, between being accepted in a circle of friends or not or between ending up in a successful marriage or not. But what exactly does interpersonal skill means and how best can such a skill be developed?
Understanding Interpersonal Skills
Personal communication skills are sometimes called "interpersonal skills" and are, in essence, a broad set of competencies that are related to people interaction. Such skills are interpersonal skills particularly the oral and nonverbal communication, listening, understanding of perspectives when dealing with people, compassion, reconciliation, and cooperation. By cultivating such skills, one can be able to better relationship skills, improve job performance and hence lead a better life.
The Importance of Self-Awareness
The process of constructing proper interpersonal skills starts with personality examination. Knowledge of the self is important; it contains strengths, weaknesses, feelings, and behaviour towards different conditions. Self- awareness also makes one conscious of how his behavior impacts the others making one make more conscious attempts to change such behaviors.
To step up your self-awareness, begin by essaying a look at how you cope with people around you. Persuade your interlocutor to change his/her mind by the way you sit or stand, speak or gesture. Moreover, one might try asking friends, work colleagues or mentors to tell him or her how they experience that person. Such feedback can be very useful to understand potential of development.
Mastering Active Listening
Interpersonal communication entails a few measures, but one of the most vital is active listening. While considering active listening one must remember that it is more than just hearing the words that are told by someone; it also involves comprehending the message conveyed and even reacting to it. In other words, active listening is a way of gaining the other person's trust, admiring them and making the connection stronger.
Imagining is part of the active listening process, and to achieve this, whites the speaker is talking, try not to interrupt or even start framing an answer. These would go a long way in acknowledging the speaker and showing the audience that one is following what the speaker is saying by using non-verbal gestures like nodding and extending eye contact. Summarize the data or information that has been delivered by the speaker and parrot the words spoken by a speaker in order to check the level of understanding an unfamiliar language and to get rid of uncertainties.

A blend of biology and technology, biotechnology has advanced greatly in the recent past decades and its applications cut across the different sectors such as health sector, farming, and ecology. With the emerging advancements in genetic engineering, synthetic biology, and that of the personalized medicine, biotechnology seems set for the sky. The next horizon in biotechnology holds potential remedies of some of the greatest problems in the world, providing solutions to mankind in health, food and the planet.
Personalized Medicine and Gene Editing
Another great anticipation in the field of biotechnology is the constant innovation of the techniques in individualized treatment. Mainstream medicine practices are generally reductionistic and take the form of the utilization of medicines and procedures for standard treatment that is applied to a majority of patients. However, personalized medicine aims at providing health care to the patient regarding his genetics, behavior, and even surrounding. Secondly, the uses of gene editing have also changed with the kind of technologies that are available in the present world such as CRISPR-Cas9, these technologies have enhanced the ways of gene editing by allowing the scientists to edit genes with high precision. It creates the possibility to genetically mend a life-threatening disease from genetic birth defects, effectively creating cures for many diseases previously deemed untreatable such as cystic fibrosis, muscular dystrophy, and some forms of cancer.
Big Data and AI in Healthcare
However, even diseases and disorders call for personalization as a tool going beyond gene-based changes. The fortune of biotechnology may be summarized by the daily incorporation of big data and artificial intelligence that can parse large amounts of genetic, clinical, and lifestyle data. Since these technologies make it easier to identify diseases that have potential to affect someone, the medical practitioners can come up with ways of dealing with the diseases most proficiently and even come up with ways of preventing such diseases when they have not affected the patient. These changes are anticipated to enhance the efficacy of the health care delivery by moving into precision medicine where treatments are not only efficient but also particular.
Biotechnology in Agriculture
Agriculture is another field where biotechnology is all set to make a big difference from the current scenario. That is the reason that with population crossing nearly ten billion in the year 2050, the menace of food security is vital. To address the challenge of feeding the growing population, biotechnology comes up with the necessary techniques of raising yields, commanding nutritional value and coming up with crops that are disease and climate change resistant. Genetic modification for example has already seen crops with better characteristics like drought tolerant corn, bollworm resistant cotton. Sometimes, such developments assist farmers to feed the ever-growing population and at the same time popularize the use of natural pesticides and fertilizers in farming.
Novel Organic Resources
As for the potential beneficial application of agricultural biotechnology in future, it may also center on the creation of completely novel organic resources. Biological engineering, a new interdisciplinary field of science, is making lab-produced meats and plant-based products to resemble animal-based products in taste and texture. These ideas might help declaim the effects of livestock farming which accounts for a large share of greenhouse exhausts, soil depletion, and water pollution. That is why as these technologies develop and more companies and governments implement them their potential for creating a better, less wasteful, and more inclusive food system on a global level can be enormous.
Environmental Biotechnology
Another rather mysterious field which could have large potential for future is Environmental biotechnology. It is worth mentioning that there are approaches toward the solution of the climate change, pollution, and depletion of resources which are afforded by the described field – biotechnology. For instance, researchers are working on bioremediation, which is the application of microorganisms with the engineering capability to eliminate oil pollution, plastic waste and other toxic substances on the ground and in water bodies. They are very effective bioremediation techniques that use the force of nature in order to try and revert some of the damage done on ecosystems. Moreover, the progress in one of the bioenergy types, in particular, biofuel based on algae or agricultural residuals, would supply novel and environmentally friendly power sources instead of fossil fuels that cause significant amounts of carbon emitter and threaten the climate change.
Biofabrication
Moreover, there are other potential and probable application of biotechnology in the future: biofabrication, it is a method of manufacturing materials and products using living cells. Biofabrication has the possibilities to remodel the way of manufacturing because it produces sustainable and biodegradable products including bio-plastics, bio-textiles and bio-constructions materials. It not only eliminates the negative impressions of conventional manufacturing solutions on the environment but also extends opportunities for innovative product development, including self-healing materials, or fabrics, which colour and texture change depending on the environment.
Ethical Considerations
Ethical issues have assumed importance in the advancement of this technology as biotechnology progresses with time. The ability to control living organisms on the gene level creates issues of potential hazards and unforeseen consequences of use of such technologies. Areas like modification of human genes, the effects of releasing GMOs to the environment and sharing of biotechnological developments to the needy, are sensitive areas that should not be left without legal regulation. In this way, both scientists, the general public and policymakers want to implement beneficial biotechnology while at the same time minimizing the adverse effects that emanate from the release of 'Frankenstein' monsters.
Conclusion
Biotechnology in the future is expected to offer great opportunities in solving some of the problems characteristic of the present-day world. Biotechnology is preparing for personalized medicine, sustainable agriculture, the conservation of the planet, and biofabrication that was previously seen in movies. Thus, it would be important for the society to continue to forge ahead into this exciting frontier with a clear and strong ethical responsibility to the benefit of mankind, the quality of health, the sustainability and equity of the world we live in.

In the current world that is characterized by a high level of competition and dynamism, diversity and inclusion are some of the core competencies in the global economy. Those companies that adopt such values not only enhance the stability of employees' work environment, but also become step ahead in their respective niches. In essence, issues to do with diversity and inclusion are not simply moral and social issues, but factors that define a company's success and its potentiality to acquire good talent.
Enhancing Creativity and Innovation
Another rather valuable value of the diverse and inclusive work environment is the improvement of the organization's creativity and innovativeness. The fact that people from different backgrounds, culture or experience work in an organization means that different angles or different approaches will be given to the same problem. An advantage of a homogenous team is that there are few different ideas being brought to the table; the disadvantage is that this increases the risk of groupthink. On this aspect, the diverse team will always be in a position to question conventional wisdom and look for new solutions, which are innovative.
For instance, a team from different cultural practices may bend a problem in different perspectives, which a similar group can miss. The very fact of such a thinking is unappreciable in fields which are most competitive and call for innovation. Alphabet, Apple and Microsoft, Microsoft and many other leading companies of the world have always given a lot of emphasis on diversity as being key to innovation and the stories of these companies tell it all.
Some New Thoughts towards Improvement of Present day staff Satisfaction and Retention
It's not a line that turns your organization into one with diverse work force; diversity is all about a situation where each individual in an organization is welcomed, valued and is able to produce to the optimum. Therefore, when the employees are with TU and committed Towards the organisation they would perceive their co-employees and supervisors as being inclusive of them. This leads to increased job satisfaction, low turnover, and better and more stable working force on the other end.
Another advantage of maintaining employees' diversity is that the companies with diverse employees are likely to be more loyal. When people think they are special and that every bit they put into the company is valued, then they are likely to continue working for that company rather than searching for another company in which to work and therefore companies will not incur the costs of having to search for other employees to employ. In addition, the policies that help in provision of equal opportunities like flexibility for work arrangements, support for work-life arrangement and the scope for career progression makes the employee engagement stronger.
Attracting Top Talent
In the current world, quality workforce is always keen on being associated with any employer that supports equality of everyone in the work place. Job seekers are more and more conscious of their choices and which company they want to dedicate themselves to and their need of finding a workplace that welcomes them regardless of their race, gender, religion or any other characteristic they might present. Firms with good Diversity and Inclusion politic will have more applicants because the pool of applicants will consist of those who fall in the diversity groups.
Also, diverse and inclusion policies may act as a signal that a company is a good place to work at for more and diverse applicants. HiPOs can enhance the image of their firms, and therefore customers' and applicants' attitudes towards such firms may be more positive. This positive image is useful in the process of sourcing for the right and quality talents, making firms to have a competitive edge in the market.
Boosting Company Performance
Studies have it that companies that embrace diversity within their workplaces usually post higher revenues than organizations that exhibit bias at the workplace. A McKinsey & Company research conducted with 2018 indicated that organizations in the leading quartile of gender diversity of the executive team were 21 percent more likely to have better profitability than their competitors. In addition, those companies and organizations in the top tunes for ethnic and cultural diversity were 33 percent more likely to outperform on profitability.
The evidence on the link between diversity and business performance can be explained by many factors. More diverse teams can appreciate a much larger group of clients and, therefore, ensure a higher level of satisfaction, as well as higher market share. Third, organizations that embrace diversity have been pointed to be having higher employee morale; thus productivity and rates of absenteeism are relatively low.
Cultivating the Right Corporate Environment
Another area that is majorly associated with firm culture includes diversity and Diversity and Inclusion. Any successful workspace that operates to its full potential must ensure that its employees feel that they are an important part of the specific identity of an organization, in other words, Diversity corresponds well with positive corporate culture. For employees to feel that they are accepted for who they are and not just put up with, then the chances are high that they will be willing to work hard and spend time on group tasks.
However, a culture of inclusion makes the employees to feel free and share some ideas as a way of solving the existing issues and coming up with better solutions. Currently, through the diversity and inclusion policies, a company can arrive at an opportunity where each employee is motivated to bring his or her best to the company.
Being Ethical and Legal
Last of all, diversity as well as inclusion is not just a strategy; it is a legal and ethical responsibility. Most of these countries have policies and legislation that compel organizations to adopt policies that do not discriminate and affirmative measures on employees. Companies that actively advise Diversity and inclusion policies will avoid legal entanglements and show they support; social justice a lot.
Also, over the years, people have become more conscious of their surrounding environment and are hence putting pressure on organizational forms to answer for their effects on the population they serve. Diversity and inclusion management can promote the relationship between companies and different stakeholders, improve the communication, corporate responsibility, and deliver the benefit of the diverse organization for the society.

Landing an interview is quite a daunting achievement within the process of searching for a job but the work is not yet done. It cannot be underemphasised that appropriate preparation for an interview can mean the difference between being offered that dream job and being rejected. Below are the tips that will allow you to be fully prepared for the next job interview and feel quite comfortable.
1. Research the Company
Irrespective of the intensity of your preparations for an interview, get time to study the company you are attending for the interview. Know how and why it works. Visit the company's website, go through the latest articles, and watch or read the social media accounts. They include their products or services that they offer, gains that they have in the recent past, and losses that they might have incurred. This knowledge will not only assist in the act of answering but also show you are a real interested member in the company.
2. Understand the Role
First, give time to read the job description and all the requirements. Determine what is expected of the employee and what kind of behaviour would be expected from the employee. The regular trappings of enrolled universities include: Enlist and examine yourself against the following standards: How do your previous years' experiences match up to these standards? It is going to be useful if you are ready to provide some references taken from your previous work and speak of your achievements that will be appropriate for the given position. This will assist you in putting more lights on how you will support the company to achieve its goals and objective.
3. Prepare Your Responses
You need to be ready to answer questions that may be put to you at one time or the other during the interview. Practice answers to questions such as:A few of the practice questions that relate to such questions are as follows;
"The two most common interview questions can be summed up by the following questions: Some of the typical questions that the participant will be expected to answer include: 'Can you tell the people some little about you?
"It's like what; what have you got to bring to the table and what do you lack at?" "
'Then ask me why I would like to be working here?'
This is an example that illustrate a case of a difficult circumstance and how it can be handled to the optimum. "
While responding to each question make sure you use the so-called STAR technique; Situation, Task, Action, and Result. They also enable the answerer to give out appropriate and related briefs that therefore emphasize on your problem solving ability and your outcomes.
4. Produce Questions for the Interviewer
Interviews are not a one way process. Make a list of intelligent questions you would like to ask the interviewer. This also proves your concern in the particular role while at the same time making you be in a position to know if you fit the company and the position. Some questions to consider include:Some questions to consider include:
Generally a question that is posed to get a candidate to expound more about the specific job is the following question:
Thus, we'd ask, 'In this position, how would success be judged?'
"There seems to be opportunities for professional development."
'Can you give me information about the team which I will be interacting with?'
5. Practice Your Interview Skills
Perform pretend interviews with a friend or relative or with your career counselor. This way is also beneficial because you are able to get more familiarized with the interview process and even on some ways it may help you develop your delivery. Pay particular attention to the manner in which you sit, talk and the quality of your responses to the questions that will be posed to you. In addition, the feedback can also be gathered interacting with a mirror or, even better, videotaping oneself.
6. Plan Your Attire
Select business and formal clothing that coincides with the organisations sector. In case of uncertainty, then it is safe to dress formally for business setting. Make sure that your clothes are clean and do fit properly on you, and the clothes should indicate the kind of job that you are going for. This is so because dressing well comes in handy with regards to creating the right first impression and also increases one's self esteem.
7. Gather Necessary Documents
It is important to come to the interview with extra copies of your resume, cover letter and portfolio or certificates. These should be stored in a professional folder or a binder or preferably on a flash drive. Carrying any of these documents shows the employer that you are organized and have done your preparation well.
8. Prepare for Technical Aspects
If the interview is in a virtual format, check if all the technology is correctly set up. For example, make sure your Internet connection is fine, your camera and if you are going to use it – your microphone. Ensure that one is conversant with the video conferencing tool being used in the interview. Select a calm and brightly illuminated area of the room for the interview and try to eliminate possible interferences.
9. Review Your Online Presence
The employers in today's world tend to analyze the profiles of the potential candidates. Make sure you update the profile in LinkedIn and make sure it is relevant to the current status of your skills and accomplishments. Anything that could be interpretative in a wrong light should be deleted on the social media profiles. An advocate online presence helps to buttresses your fitness for the position.
10. Manage Your Stress
It is normal to feel nervous during an interview and hence controlling of stress is vital if an Interview is to be successful. Use relaxation methods including using deep breaths in the mouth or even imaging. Climb into bed early on the day of the interview and arrive at the interview venue or log into the virtual interview a few minutes early. This will assist you prepare your mind before you begin the interview so that you do not get easily agitated.
11. Follow Up
Last of all, I reckon it is always a good idea to compose an e-mail to the person with whom an interview has been just completed. In the first message of the process, the applicant should make the recipient feel appreciated for being considered for the post, express interest in the said post and finally in the brief part state why the candidate in question he/she is best suited for the post. A useful follow-up can either cement the positive image the contender has developed in the employer's mind amongst other contenders for a given slot.
Thus, when you apply the above ways, you shall be in a good standpoint of getting the interview you desire and making that all important first impression.

Information security and privacy has become an important issue in today's globalised society that defines and factors into how people, corporations and governments interact. Since technology has developed and everyone's life is associated with computers in one way or the other, it is now crucial to protect individuals' data. As simple as it may sound, this convenient world that the digital age provides also comes with an added advantage of risking the privacy of ones data. It is crucial to be aware of how data privacy functions in this context when attempting to comprehend the functions of modern life.
Understanding Data Privacy
Data privacy therefore encompasses safeguarding of individual's information from being accessed and/or used and/or disclosed without warrant. Today, personal information is gathered and utilized at an unimaginable pace and augmented by technology with little regard given to whether the individual has a clue how their information is being exploited. Be it a social network, internet shopping, mobile applications or even commons home devices people are producing data non-stop, constructing individualized pictures of their lives. These countless pieces of data are helpful for business in regards to the personalization and targeting their offers, but they are also very dangerous in their impact on people's privacy if not dealt with wisely.
Risks of Data Abuse
The other concern that has time and again attracted scrutiny over data privacy is the realization of data abuse as much as personal detail is concerned. These risks include; computer viruses, hacking, theft and loss of personal data, identity theft among other risks. The most technologically advanced security systems are not safe from hacking and there are numero us instances where large companies and organization have become victims of cyber criminal and hackers and those have stolen person's data especially the financial details, health records and correspondence. Such breaches can lead to such losses as:
- Economic loss
- Reputational loss
- Emotional loss
Big Data and AI: New Dimensions of Privacy
However, with the onset of big data and more recently artificial intelligence, new dimensions to the privacy of data have come into question. They make use of big data and personal data as an input to work most of the times; this means pulling together enormous data that can reveal details of an individual's personal life. On the one hand, big data and AI are promising tools that can help businesses create new values and optimize operations, they also put questions to data acquisition, management, and utilization. The use of AI for analysing data thus means that large amounts of data (including personal data) can be used in ways that can reveal otherwise hidden patterns and trends; but it also means that personal data can again be used in ways that the individual concerned may not have thought of, or agreed to, when their data was collected and processed.
Regulations and Data Protection Laws
There are regulations to prevail these concerns and governments of different countries have enacted data protection laws to protect people's rights. In the EU, the General Data Protection Regulation (GDPR) is one of the most elaborated examples; it imposes many strict standards on how personal data should be processed by corporations. The GDPR has become a guiding law in other geographical locations with similar laws being adopted and more attention being paid to protection of personal information. It proposes the principles of openness, responsibility, and permission, which increase the role of persons in their data.
Data Privacy as Corporate Governance
However, apart from these legal approaches business all over the world are beginning to realize data privacy as part of corporate governance. Those that pay much attention to data privacy do not only cover their backs from the legal point of view but also contribute to the development of trust between themselves and the customer. As the competence develops and more and more customers are becoming aware of their rights to privacy, businesses with a vested interest in protecting personal information can stand out and dignify themselves. It is not just the legal responsibility to put in place good data privacy; it is a competitive edge if done correctly.
Individual Responsibility in Data Privacy
But on the individual level, data privacy is a subject that one must study and learn in order to use the Internet safely. Consequently, users need to be very careful in the information they provide and the service they subscribe to. This entails aspects such as:
- The users should make sure they read privacy policies
- The user should ensure that they use strong and unique passwords
- They ensure that they activate the two-factor authentication
- They should also ensure that they are careful with the permissions they give to any application or service
It may be nearly impossible to avoid all privacy risks but it is possible to greatly minimize the chance of misuse of personal information.
The Importance of Education and Awareness
Last but not the least; education and awareness is the key that cannot be overlooked in the issue of data privacy. Thus, as the technologies advances, our definition of the private is forced to advance as well. Educational programs, public information campaigns and company awareness endeavours in the training arena are critically important for enhancing privacy-awareness. Enabling people to have the information and the capability to safe-guard their own information is thus an important ingredient to making sure that the new world of faceless electronically connected people is indeed safe and secure.
Conclusion
Thus, data privacy can be considered both vital in the contemporary world and one of the key factors that define a person's relations with technology and other people. It poses problems and opportunities that have to be tackled by prevention measures on the part of regulation, of the companies, and the consumer society. Thus, focusing on data protection we could strike the balance between the opportunities that digitalization offer and threats it poses.

Looking at the modern trends in the business environment it would be wrong to consider innovation as one of the trends, but as one of the keys to success. Lack of innovation means doom for any organization since the competition is always rising and so are other factors that need consideration. Innovation refers to new ideas, goods and services, processes or ideas by which an organisation can improve its ability to deliver value to customers, achieve sustainable competitive advantage and generate adequate profits. The following article is a discussion of innovation as one of the most determinant factors of business success, elaborated through concepts of competitiveness, customer satisfaction, organizational performance, and market opportunities.
Driving Competitiveness
Creation of innovations is known to play a significant role in the degree of competitiveness of a particular firm. Wherever competition prevails, then the skill to innovate separates a business organization from other business organizations. Insofar as organisations produce new or enhanced products, they are able to stand out by creating a market or by capturing more of the existing market share. For instance, those organizations that incorporate the sector in the innovation of better products or in use the technology to enhance services provision in their companies act as the market leaders. This factor is a key source of competitive advantage and contributes not only to revenue growth but also to deepening the brand's market position.
Third, innovation entails that organisations are in positions to respond to changes in the market environment aos. The renowned business concept of 'gearing,' is another important factor that enhances business operation particularly in those industries, which are deemed to be rather sensitive; some of these industries include technology and consumers goods industries where firms that are able to make rapid strategic moves, produce unswerving products and/or services, or respond swiftly to new trends or even the consumers' demands, are likely to sustain themselves in the market. For instance, the increase in e-traders made the physical store traders transform and design web applications with improved customers' experiences. The companies that have adopted these changes are not only alive, but also have grown their market share.
Enhancing Customer Satisfaction
The two key principles of business development are keeping the customer happy, and in order to do that, introducing that element of novelty. The modern customer is a far cry from the simple, passive, easy-to-sell-to buyer of earlier yester-year. It touches on the need to find good qualities products and services through going an extra mile and sourcing for quality services from a competent sources.. Innovation helps the companies to provide solutions that would be hopeful to the targeted audiences.
For instance, the usage of data and analytics and artificial intelligence (AI) have improved the knowledge companies have towards its customers and their behaviors. This way, utilizing these findings, a business can make a product or a service offering that fits the need and wants of customers. Such level of segmentation not only results into increased customers satisfaction but also increases brand loyalty as customers would prefer to continue patronage with the brand that has met their needs.
Innovation also helps enhance ways that a business engages customers as well as how often they do so by removing barriers. For instance, the use of smart chatbots in self-service customer care help firms to undertake customer support at any time and shorten the response time. Just as with UTIs or User Interface development, change in designs enhances the websites' and the application's appeal to the internet users ultimately resulting in high conversion rates.
Increasing Operational Efficiency
Thus, in addition to innovations in front of the value chain, companies need to manage innovation inside the firm. Reconfiguring work through new processes, technologies or business models will thus allow organisations to streamline costs and consequently enhance productivity. Resource is saved for additional investment, and innovation can be done to improve the design of spaces which in the long-run increases profitability.
Automation is a classic illustration of how innovation increases the performance of operations. There is proof that by replacing manual labor with automation many tasks can eventually be completed with far less time and money. Not only does this increase efficiency in the delivery of goods and services but also reduces on human interference. For instance, the application of robots and other automation tools has transformed production line in manufacturing industries where firms are in a position to produce more units of output with cheaper set of input and at the same time deliver quality products.
A further aspect of organizational operations where innovation can reduce cost is supply chain. Blockchain added transparency in the supply chain, IoT have reduced lead time and have improved the inventory management. These innovations enable customers to meet the market demand and cut costs or waste as well as enhance the performance and flexibility of the businesses.
Facilitating Market Expansion
It is also has been established that innovation is key to market expansion. The companies that innovate are at a better vantage to venture into new fronts, it could be in product or service offering, region or targeting new customers. That is the way through which businesses may adapt to new changes and be at a position to reap from new opportunities.

The further exploration of space that has been once considered as the activity of superpowers willing to win the Moon race, has become an international affair, which significance can hardly be overestimated for the development of the science, groundbreaking technologies, and a mankind's prospective. With such future lies a head next era of the space exploration in Prospect, one that will be optimistic with collaboration, Innovation and with search of answers to set of questions that are interesting to human beings all over the world. The end of space exploration does not mean simply to reach for further place but to know and being closer to cosmos and vice versa.
Lunar Exploration
Perhaps one of the greatest features about space travel is the expectations of manned space flight to the Moon. NASA has sought to establish the Artemis programme for human lunar exploration that intends to get the first woman and man back to the moon by mid 2020s. Artemis the mission differs from all the Apollo missions of the 1960s and 70s where the goal was to demonstrate technical prowess, Artemis aims at setting permanent human presence on the Moon. This ranges from constructing a lunar base that may be used a starting post for the future missions to Mars and the rest of the solar system. Mars, the Moon, and other planets are considered suitable for the development of crewed missions with people that can stay there for a long time; the presence of water ice at the Luna's south pole, for instance, can be used as both life-supporting material and a source of fuel for very long and distant missions of probes and rockets.
Mars Exploration
To this day, the space exploration of Mars has not been abandoned and remains the final frontiers for man. Mars, the Red Planet consistently draws interest of science and the general public on account of its potential to support life extinct or otherwise. The future missions such as Nasa's Perseverance rover is currently on Mars and future human missions, will explore for trace of past life and study Martian geology and weather patterns. Planning to send humans to Mars raises several concerns, let alone matters concerning life support system, to radiation risks on the Martian environment. However, with the technological advancements and the knowledge obtained through Artemis, and other lunar explorations the development will be realized easily.
Private Enterprise in Space
Private enterprise is also contributing to space travel in a more and more important way. Various space launch ventures such as SpaceX, Blue Origin and other companies are the ones revolutionizing the technology of rockets and making space even more accessible. Elon Musk's Starship for instance is being built with an aim to transport millions of people to Mars and in effect bring the dream of space colonization to life. These companies are not only aiming at other planets but also on creating space resources like satellites, space stations, and perhaps space tourism. The commercialization of space is opening up new opportunities for scientific research, economic growth, and international cooperation.
Robotic Exploration
But beyond human missions and space technology also robots explorations are also giving us more understanding of the solar system and beyond. The James Webb Space Telescope soon to be launched will offer access to the universe and help in the exploration of star and galaxy formation as well as the detection of exo-planets in the habitable zone of other stars. Following interplanetary robots to some of the most exciting and possibly habitable destinations in the solar system; ESA's JUICE—JUpiter ICy moon Explorer. These missions are vital, as they allow collecting data that may be useful for further manned missions, as well as expanding people's vision of the universe.
International Cooperation
Out of all the articles reviewed, this one brought a very good insight into the fact that international cooperation is now a hallmark of the modern space mission. Coming to the kind of cooperation that has been discussed above, ISS is, in fact, one of the best examples that are worth mentioning. While planning for the future utilization of the ISS the necessities of low Earth orbit after the ISS is decommissioned are being discussed, this includes potenially another space station for operations in deeper space exploitations. The cooperation demonstrated through the ISS and complementary missions like Mars Sample Return mission is an efficient way of conveying the aspects that are important in the space explorations.
Challenges and Ethical Considerations
The future of space exploration is not an easy go as it has provided above challenges. These missions incur considerable expense and there has been much discussion as to the appropriateness of the allocation of scarce resources. Another issue that needs to be discussed is ethical one: particularly, with regard to possible contamination of other planets and moons with earthly life. In addition, the military use of space threatens the space in terms of it being understood as the scientific and exploratory frontiers of the world. Meeting these needs with the need to push forward and advance knowledge will be a central tension over the next several decades.
Conclusion
While people are gazing at the heavens, space research still unites people as an admirable and very audacious human pursuit. The future is thus an invigorating prospect where many more discoveries are in the offing, new technologies are on the horizon, and where horizons are thus set to be broadened. Whether it is for a Moon mission in the near future, a Mars mission in the near future or even farther in the outer rim of the solar system, the journey awaits is one of immeasurable stuffs. Humanity will undoubtedly unlock not only the next chapter of space exploration but the remaining part of textbook space history in the next few decades.

Modern life is unimaginable without the technologies that have become a relevant factor of various fields, including education. Using of technologies in teaching has developed as a process from being viewed only as an addition to be incorporated into classroom practices to being among the main foundations of the teaching and learning arenas in present times. The concept of incorporating technology in teaching and learning processes in education has shifted the manner in which teaching and learning occurs, bring the process closer to the audience.
Enhancing Accessibility and Inclusion
An important accomplishment achieved with the help of technology in education is the improvement of access and diversity. Conventional education structures present problems to learners with disabilities, those from areas of low functionality or those from low income background. These gaps have however been closed through technology whereby different needs are catered for through a variety of learning resources.
For example, online classes, e-books or educational applications allow students to have access to the quality education regardless of their location. Technological devices like Screen reader, Speech to text, Braille, and display have helped students with disability to learn easily without much difficulties. Further, due to advancement in technology, a student is able to master concepts and subjects in his or her ease and convenience at his or her own pace.
The Education Management in Announcements, Implementation of Innovative Approaches to Change Teach/ Learn Methods
The use of technology in classroom instruction has impacted the conventional techniques of teaching and learning in such a manner that has modified them into being more of an enthusiastic affair. Another advantage that has been noted is the inclusion of multimedia resources particularly the use of videos, animations, and simulations and how they have assisted in teaching since they appeal to the different modes of learning. The use of such an approach is highly effective because it aids the construction of a richer understanding of the material and the ability to remember it for longer.
In addition, through the use of technology learning has become from teacher directed learning to student directed learning. In traditional classrooms, the teacher is the resource person and students only respond to what the teacher provides them. Technology at the same time is giving students a voice in how their learning process will be conducted. Since a richer set of affordances than traditional classroom learning is available due to the internet, students are able to select areas of interest, to search and navigate through information, and to engage with peers, thus creating a more saturated and engaged classroom.
This brings us another of the revolutionary ideas that has come out of the education system: the flipped classroom model. Here, the students watch lessons on their own usually through videos at home while they do more practical, group assignments in class. This method enhances students' critical thinking and as a teacher, an individual has more time to help the learners.
Facilitating Collaboration and Communication
Another significant aspect of the learning process is that students, educators as well as parents have been able to connect in this model through advance technology. Earlier, collaboration was confined to only physical meetings with people in and around the classroom. But communication, which is one of the primary determining factors of collaboration, has elongated through the possibility of technological interaction through various forms of media.
Application like discussion forums, group chats, and collaborative tools including Google Docs enables students work in groups on projects, share information and give feedback in real time notwithstanding their location. This has not only created solidarity among students but also has been of help in preparing them for the current world of work where teamwork is a prerequisite.
To the educators, the technology has brought the gadgets that enhance communication and interaction with fellow teachers in the same school or other schools. This promotes exchange of resources in the teaching fraternity, joint forums in issues to do with curriculum implementation so as to enhance effective teaching and learning methods that will enable learners achieve their potential in the various areas of their learning.
Also, through use of technology, there has been an enhancement of communication between educators and the parent. Information technology avenues such as websites and mobile apps help parents to monitor their child's performance, updates on the assignments and grades, teachers' messages, and vice versa, tighter home-school cooperation is achieved.
That was how the idea of preparing students for the future was promulgated.
Interestingly, education technology is not only needed for improving what is currently the case regarding education, but it is also a must in order to place a wager regarding the future. Pcs literacy is not something that people could do without in the present era, it is a commodity that is needed in almost any vocation in what the society of today has come to refer to as the technological society. Using technology in teaching activities enables the educators prepare the learners for the job markets in the 21st century.
Use of technology enhances various skills such as coding, data analysis, digital content creation as well as online research among others that can be learned by the students. These skills are very essential in so many fields and whenstudents acquire them while they are in school, then they stand to benefit in the future.
Besides, through the use of technology, critical thinking as well as problem solving and creativity that is crucial in solving modern world challenges is developed. Technology makes students recognize the existing information, estimate its significance and draw the necessary conclusion, which is very important for progress and innovative activities.
Meeting the Challenges and Promoting the Responsible Use
Nevertheless, the social use of information technologies cannot be denied, and who is not aware of the difficulties connected with it. The main issues that are discussed in the context of using technology in education are – digital divide; in other words access to technology. These two can widen the gap of inequality in education hence there is need from the policymakers collectively with the educators to ensure all children get the technological input that is required.
One weakness that can be found is that people tend to have distraction and abuse the technology system. On the social networks and blogs, students can easily get carried away and spend hours watching videos, reading news and articles. Professionals of teaching and parents must go hand in hand to instill good manners regarding the use of gadgets and the internet so that the children do not spend most of their time in front of electronic devices.
Last but not the least, due to the growth in the technology, there are high expectations on educators in terms of professionalism as a result of which they need to upgrade themselves frequently. Continuous education and training are mandatory when it comes to assessment of how teachers are capable to incorporate use of technology in their teaching practice thus offering the students the best they need.

The fact with regard to understanding is that it is a great competency on individual and organizational people, & using it the people can solve all the problems uniquely. What to some people may be considered as a gift which some people are endowed with creativity can as well be noted that it is an area that one can learn. Thus, creativity requires some guidance to the frontier, the expenditure of some effort, some openness and openness to such risks as are characteristic of the frontier. Here's how you can enhance your creativity:Here is how you can expand your creativity:
1. Embrace Curiosity and Exploration
The desire to know is the root of invention. To me, when one allows himself or herself to be curious, this means that one opens a door for new experiences, thoughts or even perception to take place. You have a desire to know more and question outcomes and selected approaches to look for better solutions. This is how you are going to bring curiosity into your daily practice, which will in turn tighten the screws on your creativity. Go to new places, read articles or books on topics that you never have before, or meet people who are of a different perspective as yourself. The more an individual comes across different ideas and environments, the more the brain has feed material for the appropriate ideas when creating something.
2. Engage in Mindful Observation
Awareness is about being conscious of the environment, the act of watching things in very detail that people do not necessarily focus on. The other way of going through everyday life is observing yourself and your environment more consciously, which in turn helps to find creative approaches. If you're on a park, in a café, or watching a movie, try to look at the things at the micro level – the colors, sounds, touches, and feelings. In the long run, this will train you on how to be keen and notice things that can be used to enhance creativity in your projects.
3. I Beat the System and Bolstered My Brain: Leave Your Comfort Zone
Schedule is death for creativity, as the latter is replaced by the former, which results in more stereotypical ways of dealing with a problem. To enhance creativity it is the high time to abandon the existing practices and opt for new ideas. Repeat – change – try – look for ways to do something in a new way: for example, to get to work; select a different place for hobbies or progress in performing certain tasks in an unconventional way. When you try to get out of the normal rut and break the routine, there is a stimulation of the brain into coming up with better ideas. One has to be flexible in the approach which is one of the fundamentals of creativity and novelty facilitates it.
4. Engage in Creative Cross-Pollination
Cross fertilization is a process of merging two ideas from two different fields or areas of study so as to come up with new ideas. A large portion of the most significant inventions that have ever been made are birthed out of convergence of ideas that barely have any apparent relationship. To increase your creativity, one has to broaden his/her subject-field knowledge and to look for the connection with the topics. For instance, if one is a writer mentioning and studying architecture as a subject might help him/her develop a new technique in story writing such as how to construct a story. If you are a scientist, then perhaps learning about art may alter the way that you see the problem that you are trying to solve. The logic, which is quite obvious, is that the greater the frequency with which turning into creative cross-pollination practice, the greater ideas stock available to you.
5. Cultivate a Growth Mindset
As with the growth mindset, it also implies that there are specific skills – and, in particular, the skill of creativity – that can be improved if an individual will only persevere. Growth mindset can therefore be defined in terms of approach or perception to challenges or to setbacks or even effort in order to get to mastery. The first, and perhaps the simplest, precondition if you would like to improve your creativity – practice the development of a growth-oriented mentality. Do not fritter on the concept that is learning and that of trial and experimental so that you can avoid error if at all possible. Every painting has to be considered as a chance to learn and progress, and not as the proof of one's proficiency. The people that support the growth theory will see past such creative hitches more easily meaning that they will be well-equipped to level up their creativity.
6. Practice Regularly and Deliberately
Similar to any other aspects that are germane to a skill, creativity exercise needs to be undergone frequently in order to transform into a mastery. Choose a part of the day that you can them dedicate to such creative work as writing, drawing, brainstorming and even solving problems. The way to make your creativity better is not happy accidents, but rather intentional practice for certain aspects of creative endeavours. For instance, being a musician, one will spend time practicing on how to come up with new chords or what style of writing lyrics they want to use. If you are a designer, you probably set a goal, for example, to make a design in only one color. Generated practice aids in building up modified creative strength and is very important especially when the mind is prepared for new concept innovations.
7. Create a Creative Environment
They are to some extent a function of your environment and probably the most important factor in creativity. Organization of the workspace, and absence or presence of noise, or any form of motivation, or lack of it, affects creativity. If you want to enhance creativity, spend time on the organization of a personal working environment that you like. Get in contact with items that make one happy and that lead to creativity, this includes artworks, books, plants and anything one can think of. Obviously, one must pay a lot of attention to lighting, colors, and arrangement as these factors essentially influence one's mood and the degree of concentration. The creative place can be defined as a location that is comfortable and inspiring and makes the brain think at its greatest capacity.
8. Collaborate with Others
Cooperation is one of the most effective means for increasing creativity since people from different backgrounds and with different experience can address a problem or a task from various sides. The staffs' collaboration with their colleagues presents an instance where work can be transferred and transpire ideas at a given precinct. To increase your creative thinking, try to involve others, this could be brainstorming session, workshops or other creative related activities. Don't be precious about your ideas: be prepared to listen to others and listen to your own input. Cooperation not only can put you off the comfort zone and show you what is possible.
9. Some free time, and to some extent, vacation.
Nonetheless, creativity, like every other skill, should not be done daily; but then, it should be done quite frequently. The brain as an organ of the body requires rest, for it to work optimally; however hard you may try to devour your mind with ideas they will crop up at the most unexpected time. He removes himself by either going to the bathroom or using a break or even lunch break or even going home for the day as it rest the mind hence solutions to such problems will be better. What you get is your mind is constantly working on it even when you are not consciously trying – and ideas often come in showers, before sleep, or on waking up. It is important to be dynamic and have some time off in what one does because creativity as the definitions provided above point out is something that one has to moderate.